I recently joined NVIDIA (2025) to work with technologies that support AI Factories. This includes the Blackwell and the Hopper architectures, and platforms like NVIDIA DGX and NVIDIA HGX. I also work on observability solutions to ensure scalable and reliable AI deployments. High-speed interconnects such as NVLink and InfiniBand Networking are central to the performance and efficiency of these systems.
AI for Operations
In my previous role (2015-2024), I led the Ultra-scale AIOps Lab at HUAWEI CLOUD, wearing several hats — Director, Chief Architect, Principal Engineer, and Tech Lead — balancing strategic vision with hands-on technical leadership. My team was distributed accross Munich, Dublin, Shenzhen, and Xian. You can find more information about our work here: AIOps for Cloud Operations (2023).
Our work involved the development of the next generation of AI-driven IT Operations tools and platforms. We applied machine learning and deep learning techniques to various areas related to public clouds such as: anomaly detection, root cause analysis, failure prediction, reliability and availability, risk estimation and security, network verification, and low-latency object tracking. Our efforts fell under the AI Engineering umbrella as discussed in IEEE Software, Nov.-Dec. 2022. This field was generally called AIOps (artificial intelligence for IT operations) or ML for Systems.
In planet-scale deployments, the Operation and Maintenance (O&M) of cloud platforms could no longer be done manually or simply with off-the-shelf solutions. It required self-developed automated systems, ideally exploiting the use of AI to provide tools for autonomous cloud operations. Our work explored into how deep learning, machine learning, distributed traces, graph analysis, time-series analysis (sequence analysis), and log analysis could be used to effectively detect and localize anomalous cloud infrastructure behaviours during operations to reduce the workload of human operators. These techniques were typically applied to Big Data coming from microservice observability data:
- A Survey of AIOps Methods for Failure Management, ACM TIST, 2021.
We created innovative systems for:
- Service health analysis: Resource utilization (e.g., memory leaks), anomaly detection using KPI and logs
- Predictive analytics: fault prevention, SW/HW failure prediction
- Automated recovery: fault localization and recovery
- Operational risk analysis: CLI command analysis
We developed the iForesight system which was used to evaluate this new O&M approach. iForesight 7.0 was the result of 10 years of development with the goal of providing an intelligent new tool aimed at SRE cloud maintenance teams. It enabled them to quickly detect, localize and predict anomalies thanks to the use of artificial intelligence when cloud services were slow or unresponsive. Many of our innovation and system developments were carried out as part of the Huawei-TUB Innovation Lab for AI-driven Autonomous Operations.
Typically, the systems we develop in Munich were deployed in 93 availability zones across 33 regions in Asia Pacific, Latin America, Africa, Europe, and the Middle East. Monitoring, observability, operational risk analysis, anomaly detection, and predictive maintenance systems supported over 220+ cloud services from Huawei Cloud. If you are interested, you can look at how the various hyperscale providers (e.g., AWS, Azure, Google, Huawei, Alibaba) compared with respect to the location of their data centers here.
Technologies
Over the years, I designed and implemented various types of systems, including service systems, workflow systems, and distributed systems. As my expertise in each field grew, I authored a book for each area to solidify my understanding. Currently, I am working on a book about Kubernetes Networking.
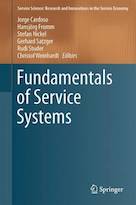
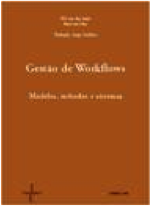
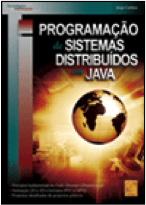
Contact
- Jorge Cardoso, PhD
- NVIDIA Munich, Germany
jcardoso [*.A._.T$] dei | uc | pt